According to conventional wisdom, artificial intelligence and specifically LLMs pose a workplace threat to humans’ more routine and repetitive tasks, while human creativity holds a distinct advantage over LLMs. Previous research on the creativity angle has been inconsistent, however, with widely mixed results.
Yet those previous studies have a major limitation, in that they are based on one task and the individual responses of LLMs such as Generative Pre-trained Transformers (GPTs) – which doesn’t reflect the fact that many people use LLMs by requesting multiple responses to one problem.
A new study posted today (5 December), co-authored at Cambridge Judge Business School, addresses this issue by comparing the collective creativity of LLMs to the collective creativity of groups of humans as measured by 13 creative tasks. These creative tasks range from social and scientific problem solving, to writing a story or an advert, to generating as many ideas as possible within a limited time period, and the tasks span 3 distinctive domains – divergent thinking, problem solving and creative writing.
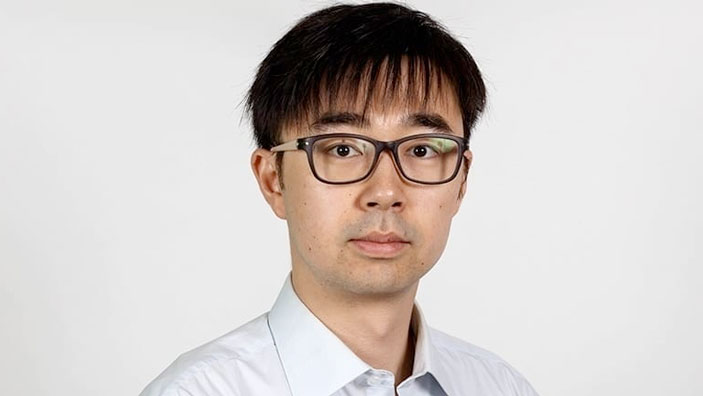
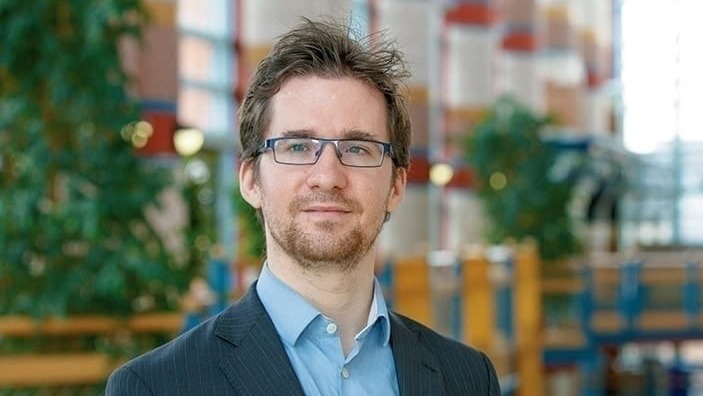
How LLMs can compete with a small group of people
The findings: “When questioned 10 times, an LLM’s collective creativity is equivalent to 8-10 humans. When more responses are requested, 2 additional LLM responses equal one extra human. Ultimately, LLMs, when optimally applied, may compete with a small group of humans in the future of work,” says the study posted on arXiv, a free distribution service and open-access archive for scholarly articles in computer science, physics and other fields.
“These findings are valuable, timely and comprehensive,” says study co-author Luning Sun, a Research Associate at Cambridge Judge Business School. “They provide empirical evidence for LLMs’ high-level cognitive capabilities in tackling creative tasks, challenging the notion that only occupations intense in routine tasks would be exposed to AI automation.”
While the notion of creativity is multi-faceted, it is generally defined as the generation of ideas and products that are both useful and novel, reflecting originality and flexibility of thought.
Comparing creative responses from Open AI GPT and 467 people
The study is based on comparing responses from 5 different LLMs including Open AI’s GPT-3.5 and GPT-4 with responses from 467 human participants from China, who completed the tasks as part of a high-stakes admission assessment for a Master’s degree. (This was an effective way to measure optimal human performance, and overcome a common pitfall of studies in which it is often difficult to get humans to put their full effort and attention into such tasks.) The responses of both humans and LLMs were rated by five trained judges independently.
Across all 13 tasks, the LLMs ranked on average in the 46th percentile against human participants, performing somewhat better in divergent thinking and problem solving than they did in creative writing, a finding consistent with previous studies.
Pooling responses yields results that reflect the modern workplace
Yet the new study’s novel look at the collective creativity of LLMs provides insight that reflects the modern workplace in which the very best ideas are sought and implemented.
“Our approach to LLMs’ collective creativity is to examine how many of the top ideas are contributed by LLMs relative to humans when their responses are pooled together,” says the research. “This is particularly valuable in real-world applications, because no matter how many reasonably good ideas are presented for a particular task, only the most creative ones will be implemented. When an equal number of top ideas comes from the LLM responses and humans, we take the number of humans as the indicator of the collective creativity of the LLMs. In other words, these LLMs can replace this number of humans in collectively generating creative ideas.”
Averaged across all tasks, the LLMs contributed about one-third of the top 10 responses, indicating that humans are collectively more likely to come up with the best responses on creative tasks.
“However, our sample included 467 humans whereas most creative brainstorming sessions in the real world include fewer than 10. We therefore analyse our results further to see what size human group would be necessary to equal one LLM when it is asked 10 times,” the study says.
By examining how many humans may correspond to one LLM in such creative endeavours, the study shows that many organisations can greatly benefit from the collective creativity of LLMs, given that a typical brainstorming session would not involve more than 10 employees. That said, in a creative endeavour that pools the global efforts of thousands of humans, such as scientific knowledge, it is unlikely that the ideas generated by LLMs would be considered the most creative.
An important milestone in showing AI’s collective creativity
The authors say that as LLM capability increases, new LLMs will almost certainly achieve higher performance, but that the study marks an important current milestone in showing that LLMs perform close to the level of a median human across a range of creative tasks, and multiple responses from LLMs possess collective creativity equivalent to a small group of humans.
The study is co-authored by Luning Sun, Research Associate at the Psychometrics Centre at Cambridge Judge Business School; Yuzhuo Yuan, Yuan Yao, Yanyan Li, Hao Zhang, and Fang Luo of Beijing Normal University; Xing Xie and Xiting Wang of Microsoft Research Asia in Beijing; and David Stillwell, Professor of Computational Social Science and Academic Director of the Psychometrics Centre at Cambridge Judge.
Featured academics
Luning Sun
Research Associate
David Stillwell
Professor of Computational Social Science
Featured research
Sun, L., Yuan, Y., Yao, Y., Li, Y., Zhang, H., Xie, X., Wang, X., Luo, F., Stillwell, D. (2024) “Large Language Models show both individual and collective creativity comparable to humans.” arXiv:2412.03151