A study on machine learning methods for bond return predictability wins the Invesco Factor Investing Prize at the Financial Management Association (FMA) Consortium on Factor Investing hosted by Cambridge Judge Business School.
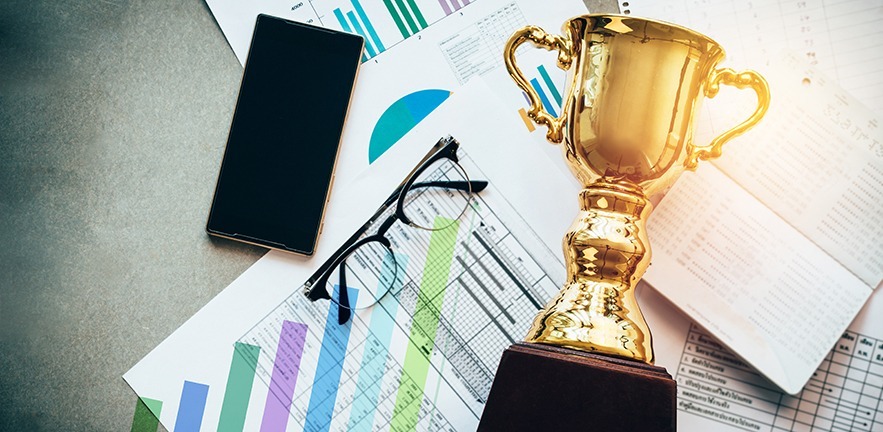
A research paper that evaluates a variety of machine learning methods for bond return predictability was nominated as the best paper contribution and awarded the Invesco Factor Investing Prize at the FMA’s 2019 Consortium on Factor Investing hosted by the Centre for Endowment Asset Management at Cambridge Judge.
The 2019 Consortium was supported by Invesco Quantitative Strategies, in collaboration with the Centre for Econometrics, Asset Markets and Macroeconomic Policy at Lancaster University.
The 4 February workshop at the University of Cambridge featured six papers on factor investing and asset management that were discussed by senior faculty members and practitioners. A summary of the Consortium is to be published in an upcoming issue of Invesco’s Risk & Reward magazine.
The best paper prize was awarded to Daniele Bianchi and Matthias Büchner of Warwick Business School and Andrea Tamoni of the London School of Economics, for their paper on “Bond Risk Premia with Machine Learning”. The prize, presented by Dr Harald Lohre of Invesco Quantitative Strategies, came with a cash award of £1,000.
The paper looked at machine learning methods such as regression trees and neural networks in order to forecast Treasury bond excess returns for different maturities. “The main results show that non-linear methods can be highly useful for the out-of-sample prediction of bond excess returns compared to benchmarking data compression techniques such as linear principal component regressions,” says the abstract of a working version of the paper.